Introduction
Streptomyces is the most common actinomycete found in our environment. These are typically gram-positive, soil-dwelling bacteria with a filamentous appearance. They have the customary earthy smell, engage in the ecological niche, and have a diversified group. Some of them have complicated life cycles. It has a very high concentration of lineage-defining guanosine and cytosine (GC), which is greater than 70%.1, 2 Streptomyces sp. has produced various drugs. Mass spectrometry and NMR-based molecular detection were frequently utilized to analyze organic compounds' bioactivities. They create several common chemical compounds under certain conditions. Bioactive secondary metabolites may have biological activities and engage in several metabolisms.3 Clinical investigations have demonstrated that these metabolites heal several pathogenic diseases. They are generally antibacterial but also antifungal, antiviral, anticancer, antihypertensive, and immunosuppressive. Streptomycetes bacteria are responsible for the production of 75% of all antibiotics. These antibiotics include ivermectin, nystatin, streptomycin, and tetracycline.4 They provide customers with an affordable alternative to more expensive procedures. They can lessen symptoms and enhance the quality of life when used alongside medication.5, 6 Streptomyces genetics play a crucial role in the treatment process. Streptomycetes' smBGCs build genes. These gene clusters synthesize bioactive compounds for new antibiotics, pharmaceuticals, and biotechnological products. Researchers can alter Streptomyces gene clusters to improve antibiotics, pharmaceuticals, and biotechnological goods. By understanding smBGC genetic components and pathways, researchers have developed new and improved antibiotics, medications, and biotechnological products.7
Streptomycetes metabolites can disrupt bacterial and fungal cell membranes to restrict growth. They disrupt metabolic pathways that allow bacteria and fungi to grow and reproduce. They can also damage the cell membrane's ability to balance nutrients and ions, reducing the cell's survival ability.8, 9 Certain Streptomycetes metabolites can disrupt critical fatty acid production, disrupting the cell membrane and reducing the organism's survival.10 First, ribosomal functional areas were blocked, which halted protein synthesis in bacteria. Tetracycline, streptomycin, kanamycin, and gentamicin are antibiotics that target the 30S ribosomal subunit. Erythromycin, clindamycin, and chloramphenicol, on the other hand, target the 70S (50S) ribosomal subunit. 10, 11 Both ciprofloxacin and novobiocin can inhibit the DNA translation process in bacteria. Carbapenems, cephalosporins, vancomycin, fosfomycin, bacitracin, and daptomycin assault the bacterial cell membrane. 12, 13 Streptomyces, a biocontrol agent, produces antibiotics that can fight dangerous aflatoxigenic fungi. They produce the antifungals streptomycin and griseofulvin. These substances can be sprayed directly on plants or used to treat the soil where the aflatoxigenic fungus is developing. 14, 15
A. flavus and A. parasiticus are species of fungi that are known for their ability to produce aflatoxins, a group of highly toxic and carcinogenic compounds. These fungi are ubiquitous in nature and can be found in a wide variety of environments. They are most commonly found in soils and plant materials, but can also be found in food and feedstuffs. Aspergillus flavus and A. parasiticus can contaminate agricultural crops such as maize, peanuts, and tree nuts, leading to contamination of food and feed products. 16, 17 This contamination can result in serious health issues for humans and animals consuming the contaminated products. As a result, the presence of these fungi and the production of aflatoxins are closely monitored by regulatory authorities. 18, 19 Polyketide synthase (Pks-A) is responsible for the initial steps of aflatoxin biosynthesis, beginning with the condensation of the hexanoyl starter unit with seven malonyl-CoA extender units to form NOR. 17, 19 They are the protein kinase that are catalyzing the biosynthesis of polyketides, a group of compounds composed of two or more molecules of ketone. NR-PKSs are a special class of PKSs that lack the characteristic β-ketoacyl reductase domain, and thus, have no capability of reducing the ketone group. This is followed by a series of highly organized oxidation-reduction reactions to produce aflatoxin. The aflatoxin biosynthesis pathway, which is highly regulated, involves the action of a variety of enzymes, including other polyketide synthases and oxygenase, which catalyze the conversion neither of NOR to the various aflatoxin compounds. Rather, these enzymes sequentially condense acyl molecules to form the polyketide chain. The resulting polyketide chain can further be modified by tailoring enzymes to form the desired product. NR-PKSs are found in fungi, bacteria and plants, and are responsible for producing a wide variety of natural products with many important applications in medicine and industry. 20, 21, 22
Streptomyces produces several bioactive chemicals that inhibit fungus, including aflatoxigenic ones. Streptomyces spp. are being studied to suppress aflatoxigenic fungi. 23 Trichoderma spp. and Bacillus spp. have also reduced aflatoxigenic fungus growth. Tetracycline kills the aflatoxigenic fungus. It has been proven to impede the growth of certain Aspergillus and Fusarium species, which create aflatoxins. 24 Tetracycline also reduces mycotoxin production by certain fungi. It also inhibits aflatoxin-degrading bacteria. It effectively controls aflatoxins in food products. 25 In this in silico study, tetracycline is taken as a ligand against the Pks-A domain to minimize aflatoxigenic effects in the human body. Tetracycline is effective at inhibiting Pks-A activity in some cell lines, suggesting that it may be a useful tool in the treatment of diseases that involve this domain.
Methods and Materials
The study design used for this research was a retrospective observational study. This type of study design was chosen due to the large amount of data available on the computational behavior of Streptomyces tetracycline against Polyketide Synthase of Aflatoxigenic Fungi. The data was collected from various sources such as published papers, online databases, and other sources. Data was collected on the effects of Streptomyces tetracycline on Polyketide Synthase of Aflatoxigenic Fungi and how it affects the growth of the fungi. Statistical analysis was then used to analyze the collected data and draw conclusions. The results of the study were then used to better understand the effects of Streptomyces tetracycline on Polyketide Synthase of Aflatoxigenic Fungi and how it affects the growth of the fungi.
PDB 3D file, structure validation and ligand retrieval
The Research Collaboratory for Structural Bioinformatics (RCSB) is a worldwide archive that stores the structural information of biological molecules that are determined by Nuclear Magnetic Resonance (NMR). 26 The 3D coordinate file 3HRQ.pdb is a polyketide synthase of Aspergillus parasiticus, which includes the PT domain. The 3HRQ is made up of two chains: A and B. For planning subsequent research, the chain A of 3HRQ was taken into mind. 20 Cello version v.2.5 was used to perform subcellular localization of the protein structure, which provides the correct placement of the protein within the cell. 27 Using PROCHECK allowed for an investigation of the quality as well as the stereo-chemical aspects of the model structure.28 The Ramachandran Plot gave the required information regarding the overall amino acid residues in the most preferred areas, the further permitted sections, the generously allowed regions, and the banned regions. It provided a better understanding of the three-dimensional conformation of the protein structure and their interactions with the backbone. The Ramachandran plot is a two-dimensional representation of the amino acid sequence in the protein. It is created by plotting the φ (phi) and ψ (psi) angles of the peptide backbone of each amino acid residue. The plot is divided into various regions that represent preferred, allowed, and disallowed conformations. The plot is used to visualize the conformations of the amino acid side chains and the interactions between them. The plot can be used to identify potential problems in protein structure and to assess the relative stability of different conformations. 29 The ligand tetracycline (CID- 54675776) was downloaded from PubChem database.
Conserved domain and binding site prediction
The protein structure has a conserved domain, which was identified through the application of public resources CDD, or the Conserved Domain Database, of the NCBI. 30 The CDD demonstrated that the iterative type I PKS of A. parasiticus has amino acid residues that belong to the PT domain area and range from 1309 to 1665. This domain is conserved across various organisms and is involved in the initiation of polyketide synthesis. Additionally, it has a "PKS_KS" motif in its sequence, which is also conserved in type I PKS. The structure of the protein also revealed a "helical bundle" motif, which is composed of two alpha-helices that form an irregular bundle, which has been found in several other proteins. 31
Molecular docking study
Computer molecular docking predicts protein-molecule interactions' three-dimensional structure. Docking systems are search algorithms with scoring mechanisms. The search technique finds the correct ligand 3D geometry (poses) in a target protein. The scoring function predicts binding affinity to measure ligand-protein interactions. 32 The most common online servers include protein structure prediction, homology modeling, and protein-protein interaction prediction. These web servers let users submit protein sequences and get predictions immediately. I-TASSER, Phyre2, and HHpred predict protein structures online. Modeler and SWISS-MODEL are popular homology models. DeepAffinity and HIPPIE predict protein-protein interactions. These free servers describe the algorithms in depth. 33, 34
SeamDock's molecular binding site module provided a 3D structural grid depiction of the target protein's active site and a graphical representation of its ligand interactions. This module allowed stereochemical studies of the ligand binding site by showing the binding pocket and surrounding amino acids. It also examined the ligand's hydrogen bonding, polar contact, and target protein conformation effects. SeamDock simplifies small-molecule docking for novices. The web server makes uploading ligand and protein data, choosing docking engines, and viewing and evaluating results easy. This simplifies difficult docking computations without requiring docking engine installation and configuration. SeamDock's NGL viewer interactively visualizes docking findings, making them easier to understand. SeamDock simplifies small-molecule docking for nonspecialists with an accessible and interactive interface. 34
Input of protein and ligand
In the first phase, the RDKit open-source chemo-informatics library converts the ligand to a pdb format. The ligand converter supports mol2, sdf, and SMILES. We use the default settings of the rdkit function MMFFO ptimize Molecule with the Merck molecular force field MMFF94 and a limit of 200 iterations to compute and optimise the 3D structure of a ligand starting from a SMILES 1D or sdf/mol2 2D representation.35 The ligand coordinates the mass centres on its place (0, 0, 0). The prepare ligand4.py from AutoDock Tools assigns atom types, computes atomic charge, and repairs missing hydrogen atoms in the ligand pdb file. Except for peptidic ligand, all torsions are active. 36 Docking programme will use the output pdb.qt file.
Docking procedure
A user can initiate docking via a button on the online form after defining a ligand structure, receptor structure, docking box, and docking parameters. Each docking programme has its own unique docking technique. The docking grid is calculated in two stages for AutoDock docking (using MGLTools prepare gpf4.py to prepare the grid parameter file and autogrid4 to compute the grid). 37 The docking parameter file is then prepared using MGLTools' prepare dpf4.py and fed into autodock 4 for processing.
Input/Output visualization
We fully incorporated the 3D viewer NGL Viewer into the web page to offer an interactive and complete 3D display of the molecules in play. 38 A 3D visualization stage lets users view computed ligand and receptor structures. Interactively explore the docking pose 3D constructions. SeamDock's NGL Viewer lets users interact with the receptor structure's docking box. The NGL viewer calculates all ligand–receptor interactions in docking outputs. JavaScript functions make it possible to show the selected docking pose from the results table interactively in the NGL stage or to illustrate a molecular interaction from the interaction tables. 29, 39
Results
We have performed molecular docking without much biophysics or computing knowledge. We used the SeamDock online server to do our molecular dock, where we performed the ligand-receptor interaction analysis. We were able to visualize the interactions in an interactive 3D environment. During the process, the molecular dock score was predicted based on the binding energies and affinity of the ligand-receptor complex. AutoDock 4 docked ligand molecules into protein-binding sites. Docking uses an evolutionary approach to determine the optimal ligand-protein binding configuration. The application uses an energy function to evaluate the ligand-protein interaction energy and find the lowest-energy conformation. Binding energy estimates ligand-protein affinity. Entropic effects account for ligand or protein conformational flexibility that could affect binding affinity. The tool evaluates solvent effects on binding affinity using empirical solvation models. The docking tool produces a 3D representation and affinity value of the docked ligand-protein complex.
Ligand input
We have used the option of sdf. file among mol2, sdf, or PDB format when it comes to the ligand input part (Figure 2). Depending on the ligand's size and complexity, ligand preparation takes a few seconds after input (Figure 1). NGL viewers show its structure in 3D. 38
Receptor prediction and docking box
A PDB file or PDB ID can be uploaded to the receptor input section (Figure 2). For example, to use chains A and E from the 3EAM structure, the user would enter "3HRQ.A" for the PDB ID, preceded by a mark point, and then "3HRQ.A" for the chains to be used. The protein, DNA, and RNA molecules themselves will survive the initial filtering process, while the amino acid and nucleic acid residues will be removed (Figure 2). After the receptor structure is ready, it is displayed in a second scenario of the NGL viewer. The ligand docking activity would be confined to the defined volume of the box. The location (x, y, z) and size (x, y, z) of the box can be defined interactively by dragging six sliders to the left of the receptor shape. As you adjust the sliders, the NGL viewer will dynamically reposition and resize the boxes to best fit the receptor structures you've selected. Options for the box's visual style include changing its colour, transparency, or wire-frame vs. ground representation.
Docking parameters and docking output
The docking parameters have been condensed down to a select few alternatives for the sake of clarity. Here we performed AutoDock, using 1.0 Å spacing, while AutoDock can be specified (0.375 Å). The mode number determines the maximum number of projected dockings within the energy range in kcal.mol−1. Here, the molecular interaction between 3HRQ.A (Pks-A) and the tetracycline found within the energy range in between -12.7 kcal/mol and -10.20 kcal.mol−1, that shows much higher potential (Figure 3). Pressing the pocket button displays the receptor surface binding pocket. There are several ways to change how the surface looks to make it more clear, such as making it transparent, changing the radius of extension, or making it close to clipping. Sticks will represent protein residues that are less than 5.0 on the ligand molecule. Protein cartoons are toggleable. The NGL viewer displays protein-ligand interactions like salt bridges, hydrophobic interactions, cation-cation stacking, and hydrogen bonds. An NGL viewer checkbox controls all interactions.
During the interaction, His1345, Cys1353, Gln1547, Asn1568, and His1345 are crucial amino acid residues that are actively involved with the tetracycline with hydrogen bonds. In hydrophobic bonds, Val1394, Val1567 and Asn1568 amino acid residues have well participated. In the interaction, His1345 acts as an acid-base catalyst in the proton relay mechanism, while Cys1353 and Gln1547 participate in hydrogen bonds with the tetracycline. Asn1568 forms hydrogen bonds with the tetracycline and also helps to stabilize the conformation of the protein. Val1394 and Val1567 form hydrophobic bonds with the tetracycline, which further helps to stabilize the conformation of the protein.
Figure 1
Ligand input overview: SeamDock offers ligand input choices. Here we used to give sdf ligand files and a SMILES specification. After ligand structure preparation, NGL will display its 3D structure.
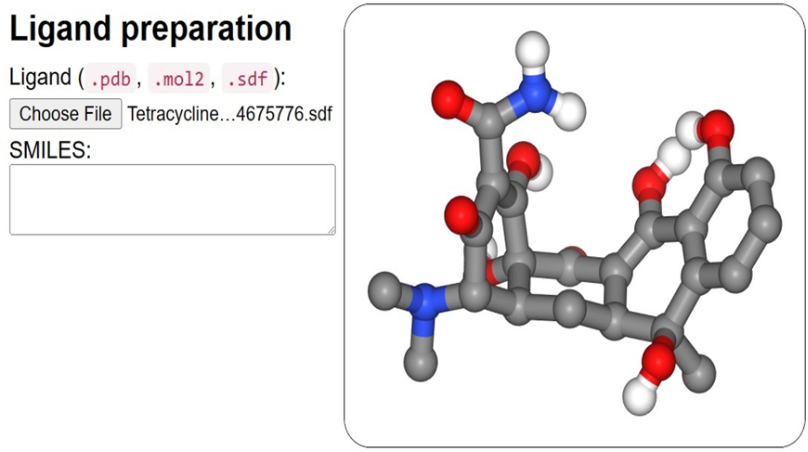
Figure 2
Receptor input overview:SeamDock has two receptor input choices. Users can enter a PDB ID or a receptor structural file with or without chains. NGL will exhibit receptor 3D structure after preparation. The six sliders just on the left side of the docking box allow interactive positioning and sizing on the NGL stage. User-customizable box and receptor look.
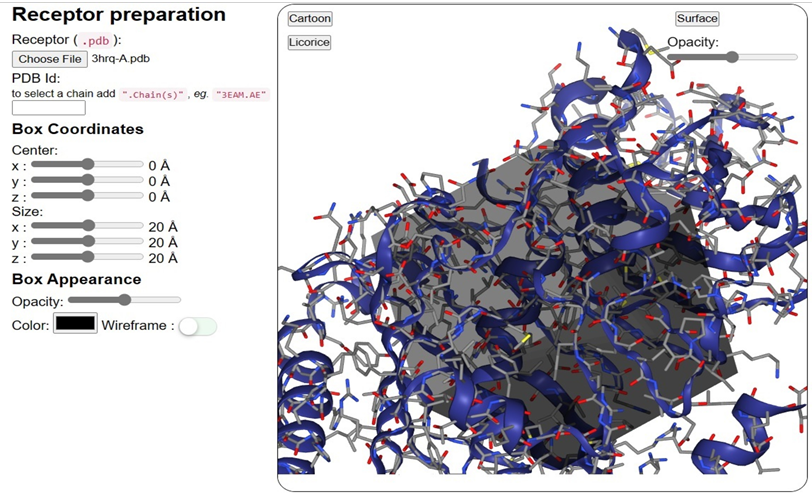
Figure 3
Explanation of the user interface for docking results (A: Pks-A-tetracycline interaction; B: atomic interaction in between the protein and the ligand; C: Surface view of the protein and the ligand interaction). When docking computation is finished, an NGL stage will show the docking posture structures bound to the receptor in three dimensions.
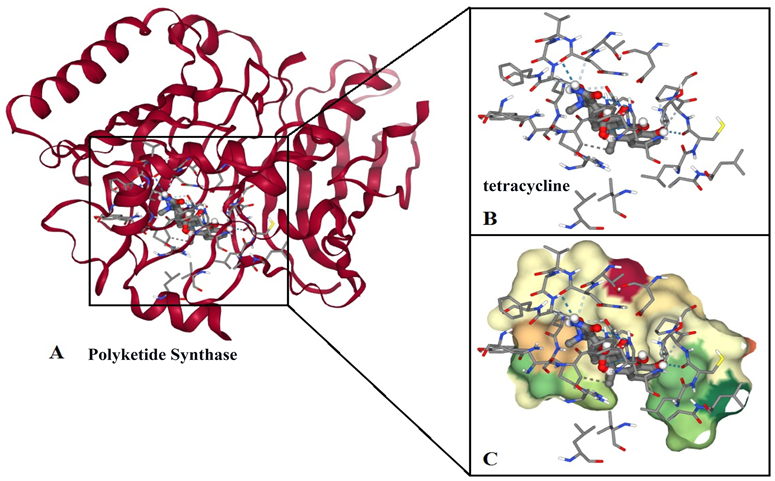
Table 1
Difference amino acid residues have involved in the PKs-A and tetracycline that show hydrogen bonds, hydrophobic bonds and weak hydrogen bonds
Discussion
To control the growth, proliferation, and biosynthesis of aflatoxin from aflatoxigenic fungi, many efforts have been taken. Here, in this computational analysis, we get the behaviour and efficacy of one of the secondary metabolites from Streptomyces sp., tetracycline, which has shown an inhibitory effect on aflatoxigenic fungi. Tetracycline is a broad-spectrum antibiotic that inhibits the growth of aflatoxigenic fungi. It works by inhibiting the production of proteins by interfering with the translation of mRNA into proteins. Its most significant binding potential is with the polyketide synthase enzyme. Although, we know that tetracycline has its antibacterial potency to control various bacterial growths, the study also has shown antifungal activities. It has been reported that tetracycline can inhibit fungal growth and it has been used to treat fungal infections.40 In addition, tetracycline can also bind to the polyketide synthase enzyme, 41, 42 which is responsible for the production of essential compounds in bacteria, such as fatty acids and polysaccharides. This binding can be used to inhibit the production of these essential compounds, thus reducing the bacterial population. Thus, the binding of tetracycline to the polyketide synthase enzyme is one of its most significant binding potentials.
The tetracyclines bind to the 30S subunit of the ribosome and prevent the formation of the 70S initiation complex. This prevents the translation of mRNA into proteins, which subsequently inhibits the growth and proliferation of the aflatoxigenic fungi. In addition, tetracycline also interferes with the biosynthesis of aflatoxin. This is achieved by inhibiting the production of enzymes required for the production of aflatoxins.20, 43, 44 By inhibiting the production of these enzymes, tetracycline can significantly reduce the production of aflatoxin. Agar Disc Diffusion Assay, 45 Minimum Inhibitory Concentration (MIC) Test, 46 Time-Kill Assay, 47 Checkerboard Assay, 40 Radial Diffusion Assay, 48 etc are some of the other methods available for checking the antifungal property of tetracycline. These all measures the susceptibility of the fungus to the antibiotic by observing the inhibition of growth around a paper disc soaked in a solution containing the antibiotic. In conclusion, tetracycline is an effective secondary metabolite that can be used to control the growth, proliferation, and biosynthesis of aflatoxigenic fungi. It works by inhibiting the production of proteins and interfering with the biosynthesis of aflatoxin. Therefore, it can be used as an effective tool to control aflatoxin production.
Further studies should investigate the efficacy of tetracycline in vitro and in vivo. In vitro studies should focus on the effects of tetracycline on bacterial cells of varying antibiotic susceptibilities. Proper controls should be implemented to measure the effects of tetracycline in different concentrations and lengths of exposure. In vivo studies should utilize animal models to measure tetracycline's effects on various bacterial infections. The models should be designed to assess the effectiveness of tetracycline in various doses and for various lengths of exposure. Additionally, the studies should measure the impact of tetracycline on bacterial resistance and the potential for side effects in animal models. Additionally, further studies should investigate the potential for tetracycline to be used as a therapeutic agent for aflatoxin-related diseases. Finally, additional studies should explore the long-term effects of tetracycline on the microbiome and its potential for cancer prevention.
Conclusion
This study of the computational behavior of Streptomyces tetracycline against polyketide synthase of aflatoxigenic fungi could be useful for pharmaceutical companies in their efforts to develop new antibiotics. By understanding the interactions between the Streptomyces tetracycline and the polyketide synthase of the fungi, pharmaceutical companies could develop new antibiotics with greater efficacy and specificity. Additionally, this research could provide insights into the development of new treatments for aflatoxigenic fungi and other related diseases. Finally, this research could be used to develop new strategies for controlling the spread and production of aflatoxins. This Streptomyces tetracycline can be used to study the computational behavior of the polyketide synthase of aflatoxigenic fungi. This can be utilized to gain insights into the molecular mechanisms of these fungi and to develop strategies for controlling their growth and spread. Additionally, this research can be used to predict the effects of different environmental and chemical factors on the expression of these fungi's polyketide synthases. In addition, this research can also be used to develop new compounds that could be used to improve the efficacy of drugs used to fight infections caused by aflatoxigenic fungi.